In support, copilots are not the answer
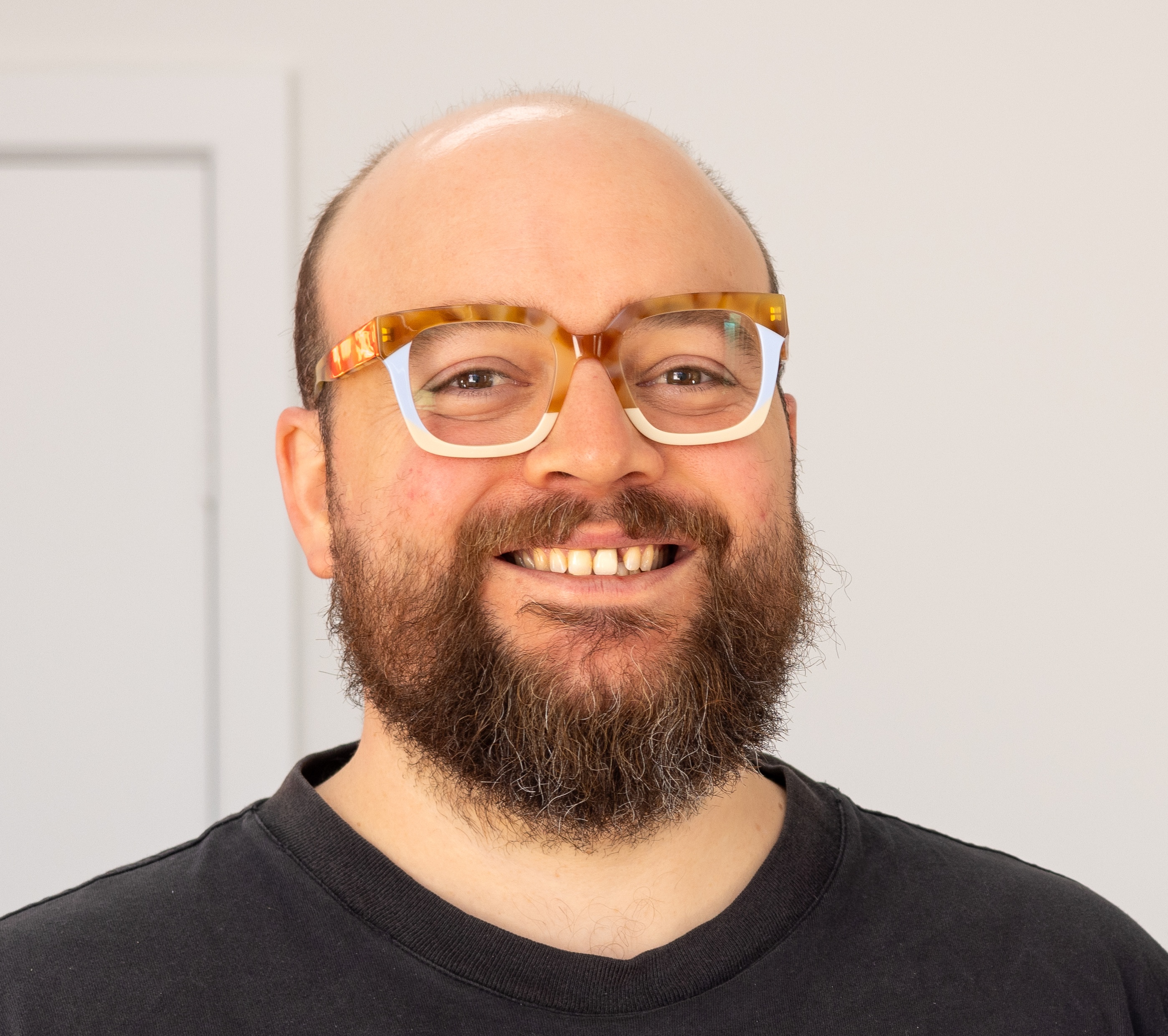
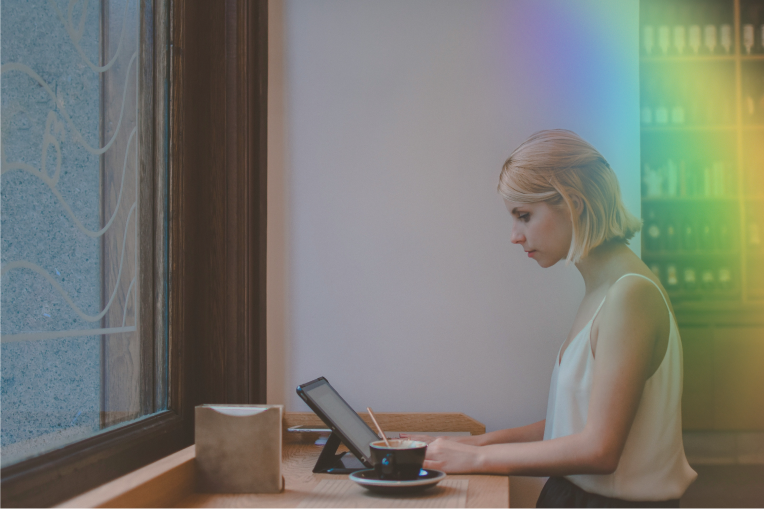
AI "Copilots" are not the answer for customer support. They're an admission of defeat by unambitious vendors and cautious, under-informed buyers. The logical case for them is weak, and so are the results they produce. Companies seeking to buy copilots today should reassess.
This post will be a little longer than usual because this topic is complex and I want to take the time to lay out my thinking. Here’s the basic thesis:
- A capable copilot is a capable pilot
- A capable copilot offers only marginal efficiency gains
- An incapable copilot may be worse than nothing
- As a result, using a copilot as a stepping stone to full autonomy isn’t effective
- Vendors are selling copilots because buyers are understandably afraid of AI
- The better solution is high quality AI agents with robust testing
I’ll walk through and unpack these points.
A capable copilot is a capable pilot
When solving support tickets, the requirements of a capable copilot system are indistinguishable from a pilot system that works autonomously. It has to understand the customer’s intent, map that intent to reference material or standard operating procedures, then gather information and execute a response.
But it’s possible the benefit of a copilot is human review reduces the risk of errors, meaning you can get the efficiency benefits without accuracy risks. That doesn’t stack up.
A capable copilot offers only marginal efficiency gains
For the sake of argument, let’s assume for a moment that a copilot always produces good quality output. Even then, it has two fatal drawbacks.
First, because copilots still require human operators to handle every ticket, any improvements can only be marginal. Erik Brynjolfsson from MIT and co-authors demonstrated this in a high quality paper released last year, finding a customer support copilot system improved tickets handled per hour by a mere 14% on average.
So for a business growing >70% year on year, implementing a copilot offsets one quarter of headcount growth. For a business growing 30% a year, it buys six months. Further, it doesn’t address the other element of scaling: responsiveness to unexpected ticket volumes. A team that’s 14% more efficient will still get swamped by an unexpected surge in ticket volume.
We’ll acknowledge that, for a very large business growing (say) 2% year on year, a 14% efficiency improvement can be useful. But most businesses aspire to grow a lot faster than 2% per year, and should aspire to get more than 14% out of AI.
Finally, it’s worth considering the human toll of the copilot success case. We’d argue the better the copilot is, the more the job becomes alienating: human agents are reduced to nothing more than rubber stamps. If you thought today’s customer support jobs could be mindless and alienating, imagine sitting like a battery hen clicking “send” on AI-drafted responses all day.
An incapable copilot may be worse than nothing
We’ve been assuming that the copilot is always good. But we know it likely isn’t - otherwise it could be operating independently.
In the more realistic case, where the copilot is correct and incorrect in some hard-to-estimate proportion, the situation gets much harder. Now the assumption of copilot efficiency rests on the idea that humans will correctly identify when the AI is wrong, and that they’ll find fixing its errors (and rubber stamping its wins) faster than just writing responses themselves.
There is good reason to be skeptical of this. First, when AI systems are wrong, they’re often overconfident and wrong. They output plausible sounding, confident and incorrect messages. Agents will not quickly and easily be able to see through the AI BS, and they won’t judge AI quality flawlessly.
So the true rate of AI assistance will be the portion of tickets the copilot gets right multiplied by the portion of those tickets the humans correctly assess as correct. So a 70% accurate model that’s correctly assessed 70% of the time is only leading to correct responses 49% of the time.
The cherry on the sundae is that the human review blunts the incentive of vendors or buyers to get the AI’s accuracy up. It reinforces the common support antipattern of throwing people at a problem instead of landing the right technology solution.
As a result, using a copilot as a stepping stone to full autonomy isn’t effective
Many companies are interested in copilots as a way to tiptoe toward deploying autonomous AI agents. But we think embracing copilots is a side quest, not a first step on the autonomy path. As above, they select for different system and vendor capabilities, and different human <> AI interaction models.
Our prediction is that the copilot moment in customer support won’t last. The copilots will – at best – generate marginal improvements. The companies that embrace agents will see step changes. So it’s only a matter of time – perhaps not much time – until companies that embrace copilots move away from them.
As a company we don’t believe that doing the easy thing – making copilots – is preparation for doing the hard thing – making autonomous agents. So in theory a copilot company will have a leg up in transitioning their users to agents, but in practice the original copilot sale should rightly erode trust, and will distract the companies from building autonomous agents.
Vendors are selling copilots because buyers are understandably afraid of AI
Despite these logical and empirical issues, lots of vendors – even newer startups – are selling copilot solutions. They’re doing it because buyers demand it. The majority of companies we talk to ask about a copilot on the first call.
This demand is not unreasonable. Copilots superficially seem like a safe way to start experimenting with AI, and are a lot easier to contemplate and introduce to an existing human team.
But one of the challenges in B2B software is selling value to the corporate customer while still satisfying the needs and goals of the buying user. Since copilots won’t deliver real value, we’ve made the choice to invest in educating buyers about alternate approaches, instead of selling them what they’re asking for. This matters because it dictates the amount of improvement they’ll be able to generate and show to their leadership when renewal rolls around.
The better solution is high quality AI agents with robust testing
Okay, so my choice is to use a copilot which doesn’t ultimately help, or take a huge risk on an autonomous AI system? No. The solution to the dilemma is to embrace autonomous agents incrementally and with effective testing and evaluation.
Lorikeet’s AI agent does not aim to draft responses for or to send responses to all tickets. Instead it’s designed to be trained to deal with specific issues, and to leave everything beyond its training alone. This allows our customers to train and test the AI incrementally, building confidence over time but driving step change improvements in efficiency as the agent takes on more and more volume autonomously.
By focusing on how we help customers launch autonomous agents, we’ve been drawn to make the agent better, and to build an industry-leading suite of testing, evaluation and rollout tools.
At the same time, deploying autonomous agents provides a much more interesting and high leverage role for the humans on the support team: configuring, deploying, testing and iterating on these AI agents.
See Lorikeet in action
Companies choose Lorikeet because our AI agent can do more than any other available. We'd love to show you what we can do for you.