Latest
|
Macro Economy
Latest
|
Consumer Finance
AI
|
Latest LLMs
CX/CS
|
Fintech
Latest
|
AI Infrastructure
Enterprise
|
ROI of AI
AI
|
Ethics & Safety
Latest
|
Politics & Policy
AI
|
Enterprise AI
AI
|
Big AI
Latest
|
Consumer Banking
Latest
|
Fintech Funding
AI
|
AI in Fintech
CX/CS
|
Fintech
AI
|
Health Tech
AI
|
AI Governance
Latest
|
LLMs
Latest
|
Fintech
AI
|
Open Source
AI
|
AI Security
Enterprise
|
Cloud Security
Latest
|
Macro Economy
Enterprise
|
Enterprise Solutions
AI
|
GRC
AI
|
AII Adoption
AI
|
AI Ethics
AI
|
Healthtech
CX/CS
|
AI in CX
AI
|
Quantum Computing
AI
|
Cybersecurity
Latest
|
Healthtech
CX/CS
|
AI Adoption
AI
|
AI
AI
|
Safety and Compliance
Latest
|
Big Tech
AI
|
Consumer Tech
AI
|
AI Ethics and Risks
CX/CS
|
AI
Enterprise
|
Data and Privacy
Latest
|
LLMs
Latest
|
Banking and Blockchain
AI
|
Healthtech
Enterprise
|
AI in the Enterprise
AI
|
AI Risk and Compliance
AI
|
AI Arms Race
Enterprise
|
AI
Latest
|
LLMs
CX/CS
|
Compliance
CX/CS
|
Great CX
CX/CS
|
CS in Blockchain
AI
|
AI News
Enterprise
|
AI
|
CX/CS
|
CX/CS
|
AI
|
CX/CS
|
AI
|
AI
|
Enterprise
|
AI
|
CX/CS
|
CX/CS
|
Enterprise
|
Enterprise
|
Anthropic warns against blind trust in reasoning models
.jpg)
⋅
April 10, 2025
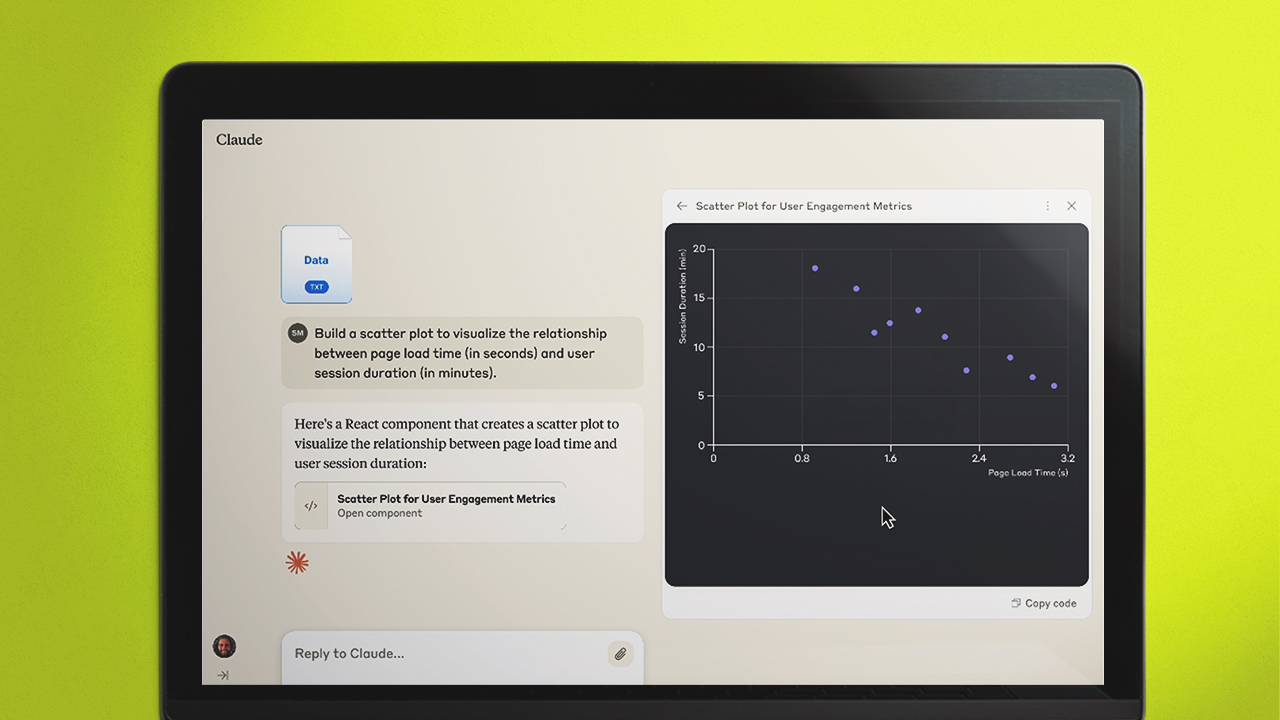
Key Points
Anthropic warns businesses against taking AI-generated reasoning at face value, highlighting potential inaccuracies in reasoning models.
The AI lab's research suggests that "chain-of-thought" prompting techniques can produce plausible but incorrect outputs.
Businesses are advised to pair AI capabilities with rigorous system design and human oversight for effective deployment.
As generative AI capabilities accelerate toward feature parity and tool costs continue to fall, Anthropic has issued a timely warning: businesses should not take AI-generated reasoning at face value.
Breaking the chain: In a new research paper and accompanying blog post, the AI lab behind Claude cautions against blind faith in reasoning models—especially those using "chain-of-thought" prompting techniques. While such models can appear to "think" through problems step by step, Anthropic's findings suggest they often produce plausible but ultimately incorrect outputs without any actual logical grounding.
The proliferation of enterprise AI: This matters more than ever as AI tools become easier and cheaper to access throughout the enterprise. With advanced reasoning features increasingly commoditized across platforms—whether from Anthropic, OpenAI, Google, or Meta—the focus is shifting from access to impact. Lower cost and friction mean engineering teams can now spend more time optimizing AI for business outcomes, rather than wrestling with procurement or integration.
Ubiquity doesn't equal quality: But Anthropic's research underscores a crucial point: better results will only come with better oversight. Just because a model uses reasoning-like structures doesn’t mean it’s engaging in real reasoning, the company says. Anthropic found that reasoning chains often reflect training data biases rather than true logical inference. In some cases, these outputs can even create a false sense of trust in a model’s reliability.
Formula for success: For businesses deploying AI agents to automate decisions, this raises both ethical and operational flags. While the technology may now be widely accessible, Anthropic's message is clear: real value comes from pairing AI capabilities with rigorous system design, human-in-the-loop oversight, and clearly defined governance mechanisms.